There’s no question that the AI industry is moving fast and the available tech is proliferating—and with all the activity comes hype, skepticism, anxiety, and curiosity. For our part, we’re interested in a pragmatic take, so we’re checking back in to understand what and how developers are really building with AI—and what’s actually working for businesses.
Are we getting out of demoware and commoditized models and into real use cases and real ROI? Are we thriving in this age of the chatbot? Just how functional is the average AI stack?
We asked ~750 tech folks—including developers, data teams, leadership, and others across technical roles and industries—to share their takes. Let’s have a look.
Use the table of contents on the left to navigate through sections if you want to skip ahead.
An updated look at AI sentiment
In our 2023 report, respondents took a measured view of AI, with most considering it slightly overrated. That hasn’t changed much in 2024 so far:
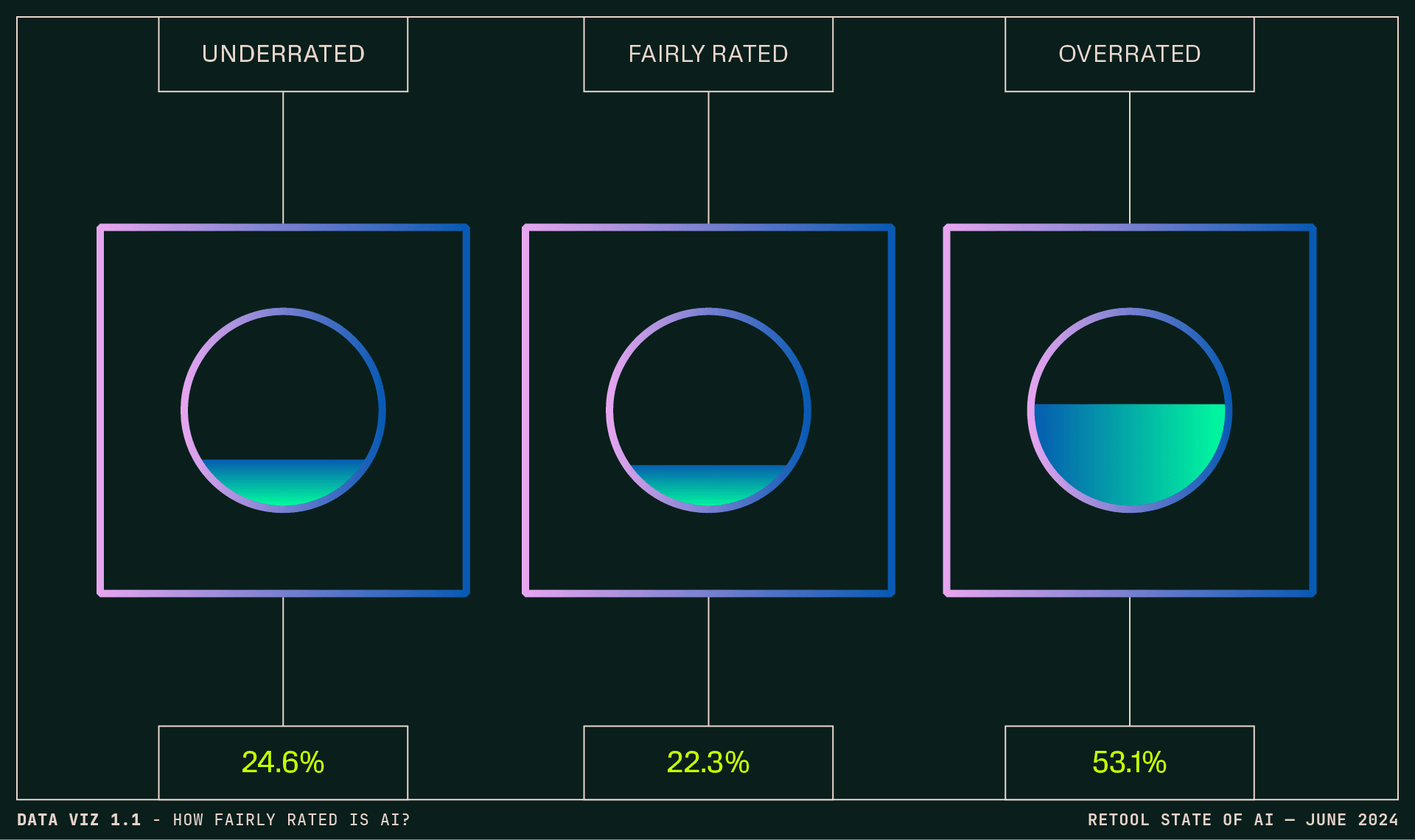
And it holds true even when breaking down sentiment by role, with ratings averaging in the 5s (“fairly rated” to slightly “overrated”) for all levels of seniority. After the hypestorm of 2023 (which, one could argue, made it hard for anything AI to meet expectations), a more clear-eyed view seems to be emerging of AI’s relative immaturity, current limitations—and big potential.
In the write-in responses, we saw a sense that the aggressive hype has temporarily obscured the true potential of AI:
- AI is being shoehorned into products without really adding value. (Anyone reminded of “we rewrote everything in $HOTLANG”?)
- “AI” is being used as a catchall for machine learning, LLMs, and automation.
- AI is being treated as a magic hammer, including in cases where conventional programming would suffice or outperform AI, without having to contend with tedious prompting and hallucinations.
It seems there’s still a lot of chaff to sift through to uncover genuinely useful applications of AI for everyday technical and business use cases, but respondents were optimistic that the utility and breadth of applications for AI will emerge.
In a word (or several): when it comes to actionable AI, this is just the beginning.
While a dominant media narrative around AI is that its adoption and utility are surging and it’s taking over the world, the reality is, for now, a bit more moderate.
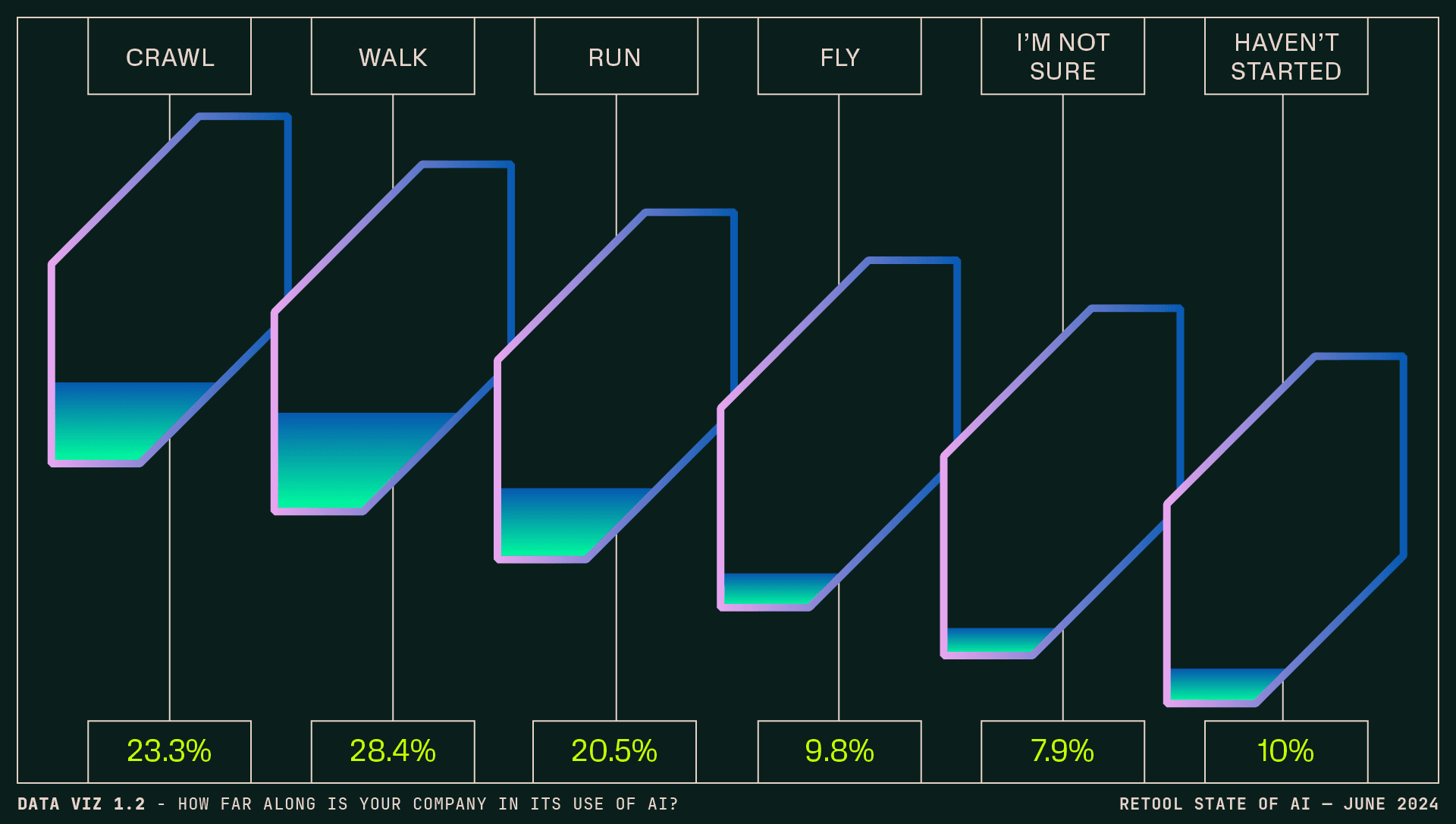
Maybe everyone’s using it, but most respondents were clear that they were a long way from the top. Plenty of respondents rated their company well—about 30% consider themselves either “running” or “flying” when it comes to adoption, and respondents in the consulting (46%), real estate (46%), and consumer goods sectors (37%) ranked most highly. But, overall, those who feel they’re leading (aka flying) are down from 13.4% in 2023 to 9.8% in 2024.
100% of respondents in the materials sector reported lagging maturity here (albeit this was comparatively low sample size); 80% of respondents at nonprofits said the same.
Moreover, the progress indicated by respondents is largely not transformative (as reporting on the outliers might have you believe)—or at least not yet, with the impact of those use cases rated an average of 6.7 out of 10. As we get more clear on the obstacles and challenges around adoption, reality checks in.
Across all roles, teams, and seniorities, only a small percentage (4.5%) of respondents felt their company is overinvesting in AI, with a fairly even split between “just right” (42%) and “not enough” (40.5%).
Going a layer deeper, hands-on leaders—directors, managers, and VPs—want that investment more than ICs do.
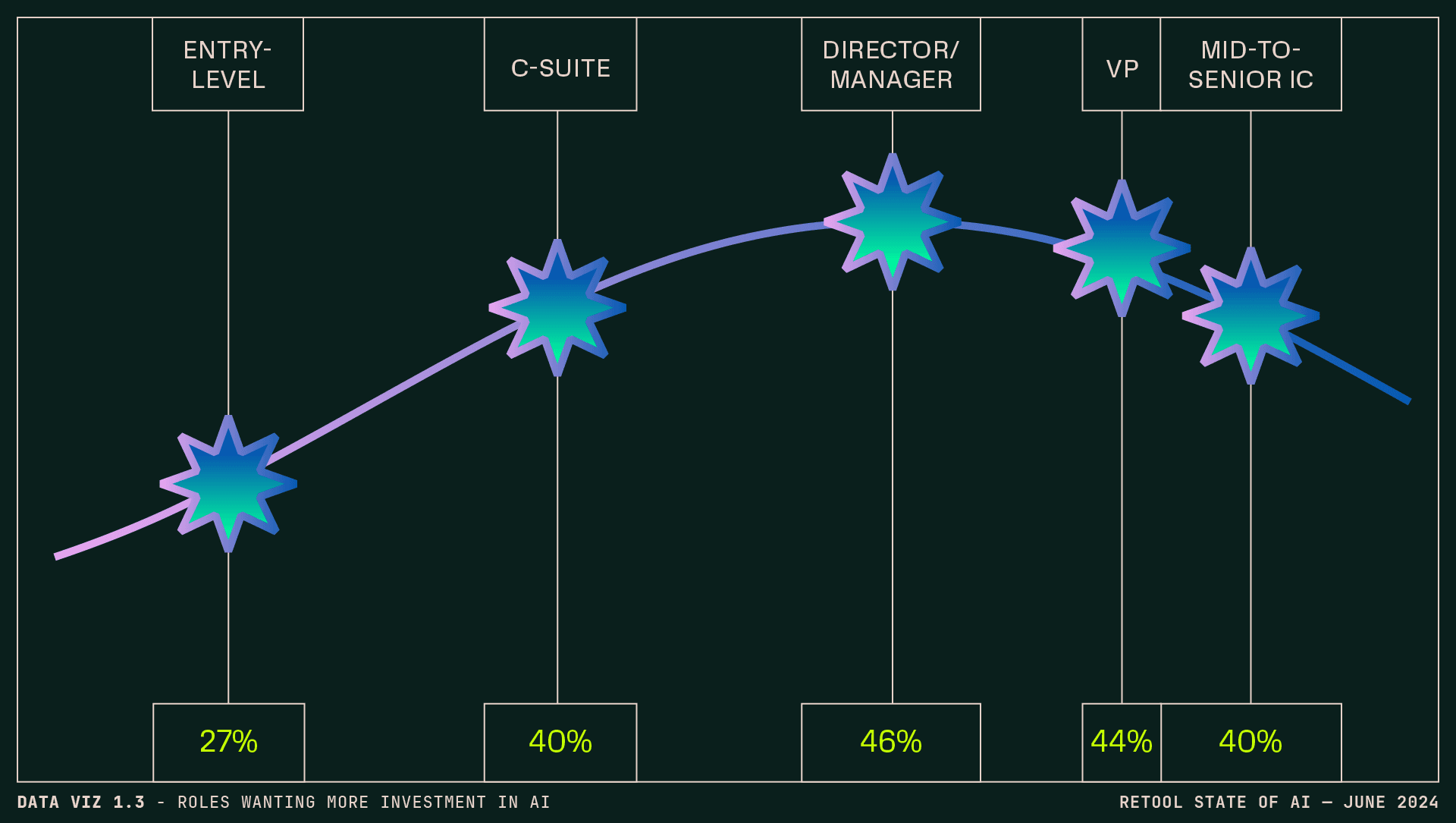
By company size, those with 50–99 employees were most strongly inclined toward more AI (50%), followed by enterprises over 5000 (45%). (All other sized companies ranged from 33–42%, with no linearity.) Of technical teams, IT workers were most bullish on more investment in AI (49%). Our friends on the design teams were less convinced (33%).
Almost three-quarters of respondents use copilots and other AI tools (e.g. ChatGPT, Claude, etc.) in their work at least every week, with 56.4% nearing daily use. At the smallest companies (1–9 employees), these daily numbers spike to 72%, followed by 59% at companies of 10–49. (While most other company sizes hover within a couple points of 50%, at companies with 1000–4999, this dips to 43%.)
By role, Product and Engineering are leading daily(ish) adoption at 68% and 62.6% respectively, while Design trails at 39%. (Our AI-bullish IT friends are right in the middle of the pack, at 50.1%.)
Overall, a strong majority of respondents who use copilots or other AI tools at work report increased productivity from doing so:
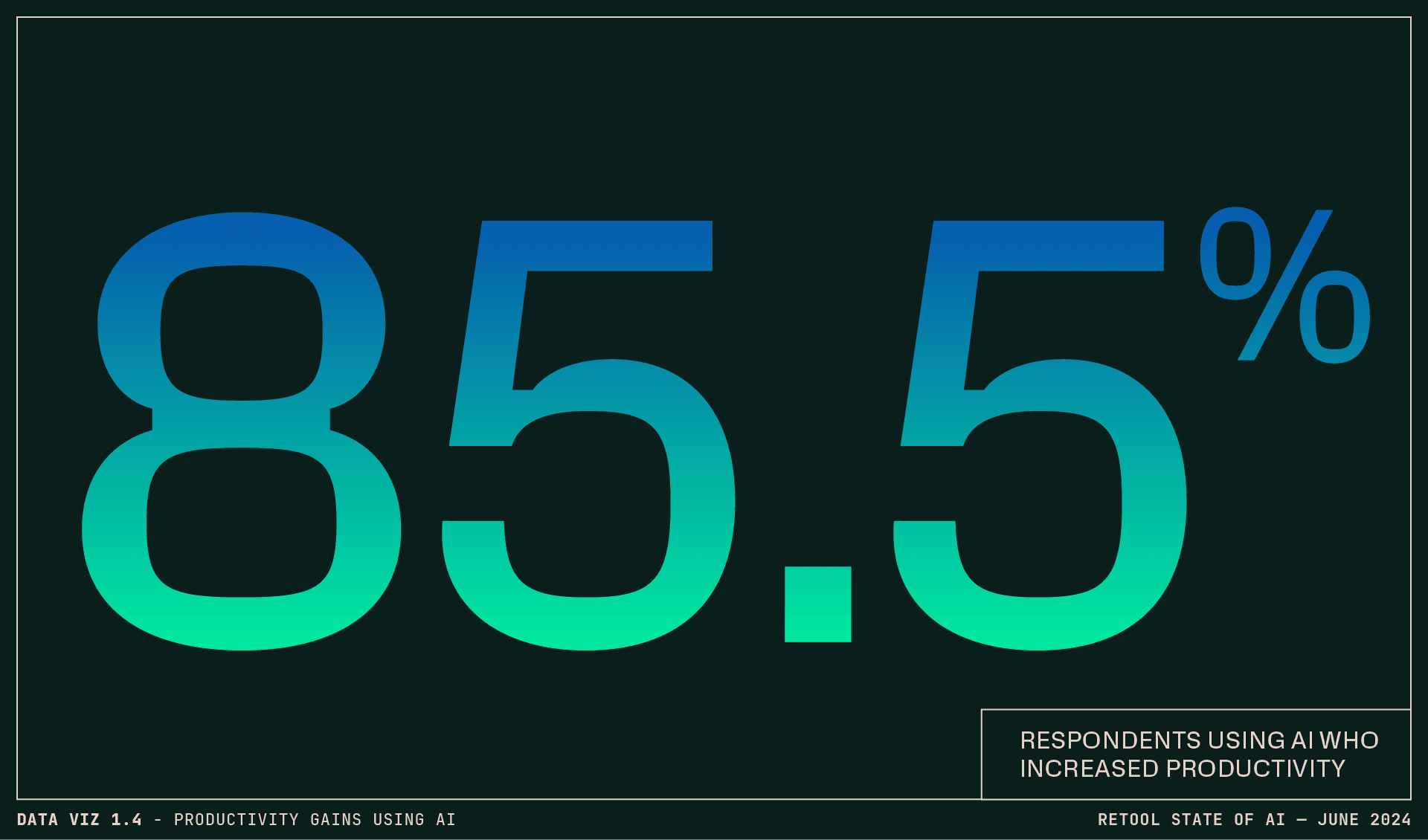
It also plays out that the more you use AI tools, the more likely you are to find them valuable: 64.4% of daily users report significant productivity improvements, versus 17% of weekly users and 6.6% of occasional users. (Fair enough?)
Last go round, we asked the kind of weird thing out loud: Are you secretly using AI at work? And quite a few people were (34.4%). This time, we wanted to see where this was trending and better understand what was going on. So let’s break it down.
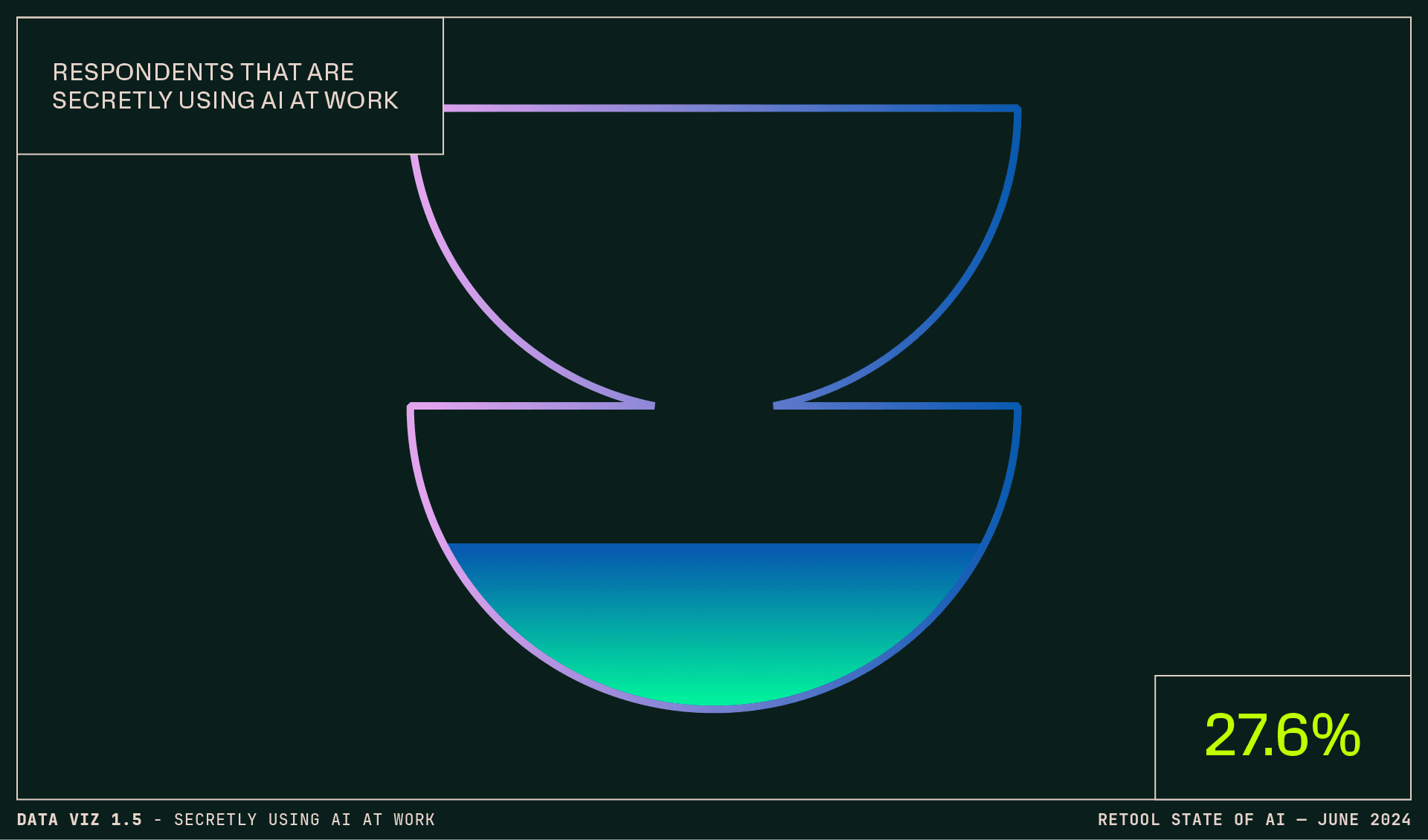
A higher percentage of respondents are allowed to use AI at work today than they were ~6 months ago (64.2% vs 54%), but more than a quarter (27.3%) of respondents are still using AI in secret at work. This is trending down from 2023, but given the proliferation of AI features now available throughout a business’s stack, somewhat surprising. So, why be cloak and dagger about it?
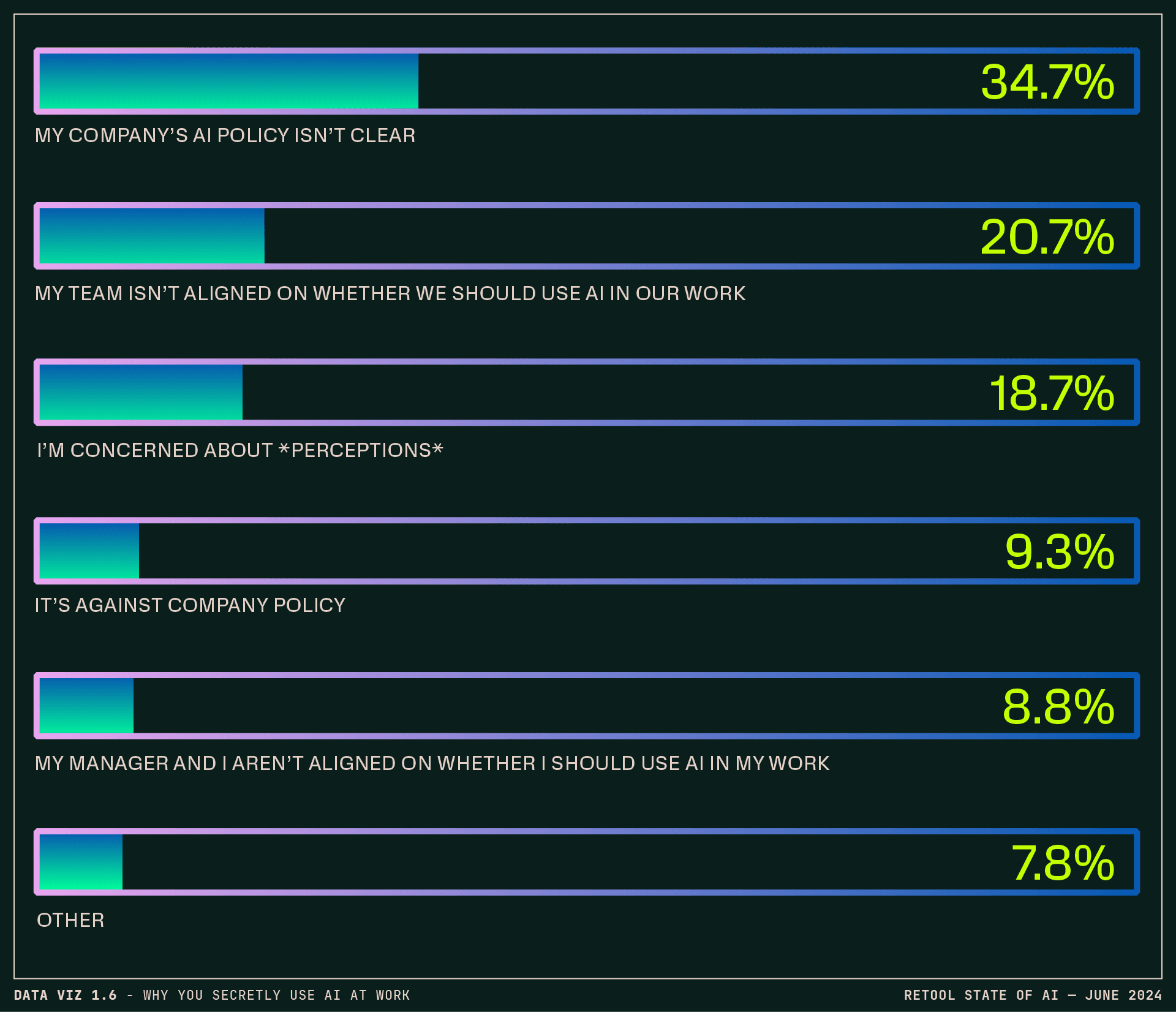
Only about 9% of those secretly using AI are doing so directly contrary to company policy—other motivations are around internal alignment and perceptions about using AI, or a lack of clarity on policy. An overall uptick in interest in using AI for work since last year (which may even be for arguably benign use cases like summarizing meeting notes) together with regulation not keeping pace with adoption could be creating a bit of a murky environment...
(On that note, 62.9% of respondents are paying at least some attention to emerging AI regulations and policy.)
If you’ve been on the internet lately, you might say the takes on whose job is likely to disappear due to AI are hot. As “everyday AI” matures into real use cases and ROI, should we really be worrying about human versus machine? Will software engineering become obsolete?
Our respondents had some thoughts. While a well-grounded 15.3% don’t think anyone’s really at risk of being replaced by AI (new technology = more demand = more need for engineering resources, areweright?), 45.7% rated entry-level ICs as most at risk of replacement by AI. In the write-ins, things get more nuanced—change is a reasonable expectation. After all, if entry-level ICs are replaced, where would the mid-to-senior ICs come from down the line when the world still needs more software than it can build? 🤔
Middle management takes second place (13.2%), but at companies where middle managers are mostly supervisory rather than strategic, it could be easy to imagine those functions being automated to some extent. (On the other hand, freeing up time through automation might allow those middle managers to be more strategic…!)
Senior ICs and executive/senior leadership are seen as low risk.
And relatedly (or not) executives’ enthusiasm in adopting AI has been strong: C-suite respondents were the biggest daily users of AI at ~72% (other roles range from ~45–56%). They also reported the highest impact and productivity increases versus other roles, adding to the idea that AI is less of a threat than a tool. (Our 2023 report found that C-suite and VPs had the biggest expectation of AI changing their roles, so investing in upskilling with AI is consistent.)
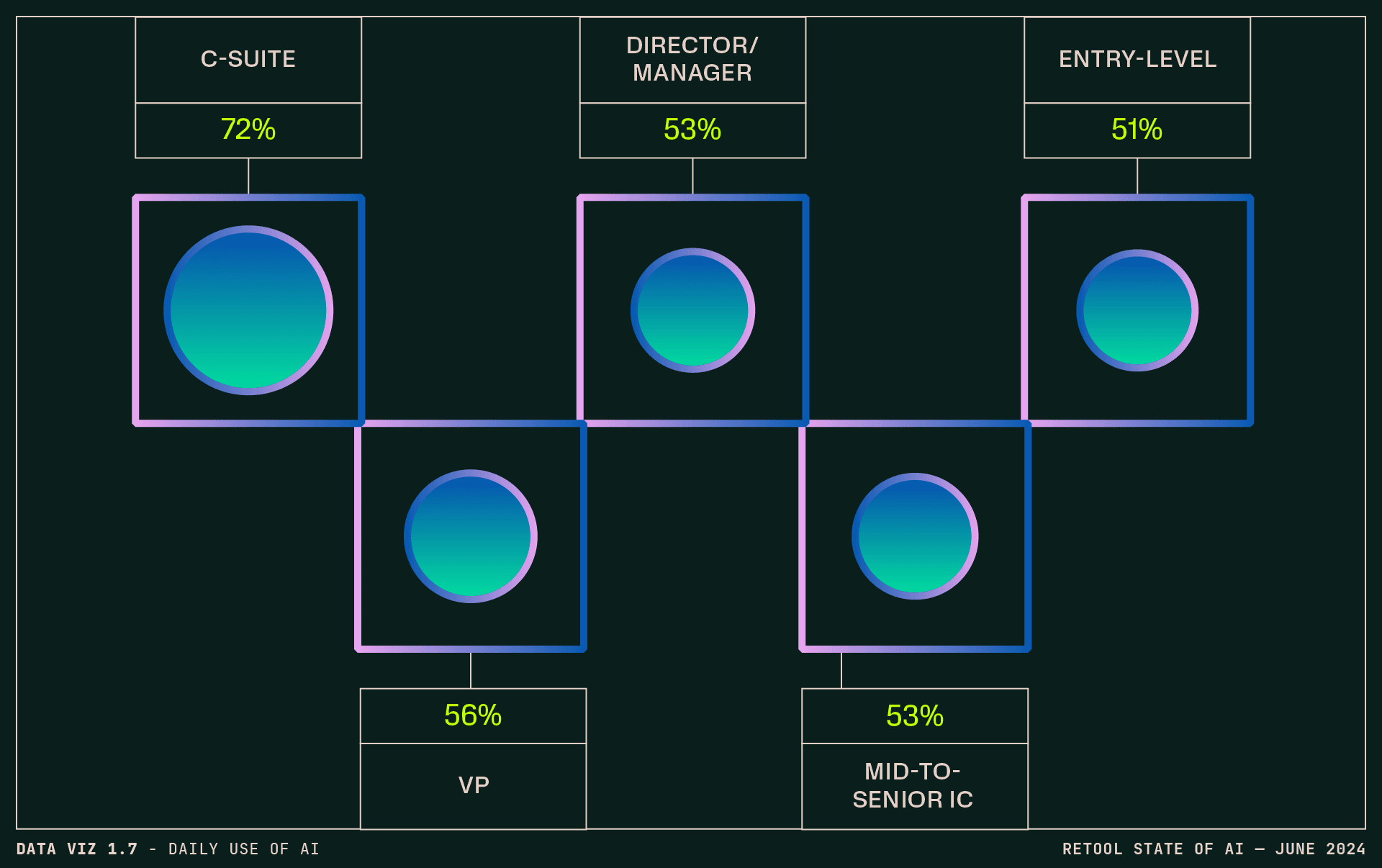
Perhaps this all speaks to an opportunity: using AI as a lever can help both leaders and ICs create a moat for themselves. The competition, as it were, is likely not between humans and AI—but between humans and humans using AI. (A notion supported by numerous write-in responses!)
Real AI use cases and ROI
You get an AI-powered chatbot, and you get an AI-powered chatbot…
Just over half of respondents (55.1%) have either built an AI-powered chatbot or their company has. (16.8% had built three or more.)
Despite (or because of) their proliferation, we heard a little chatbot fatigue from respondents lamenting that these are the extent of all new “AI” features or products. While anecdotally, chatbots seem to dominate public-facing AI apps (including RAG-enhanced support bots, like the one rolled out to great fanfare by Klarna), these are likely the most visible because there’s a lot of them, and they’re the easy use case to implement for now. It’ll be interesting for us to watch this develop and see if the luster—or fatigue—fades.
A prediction:
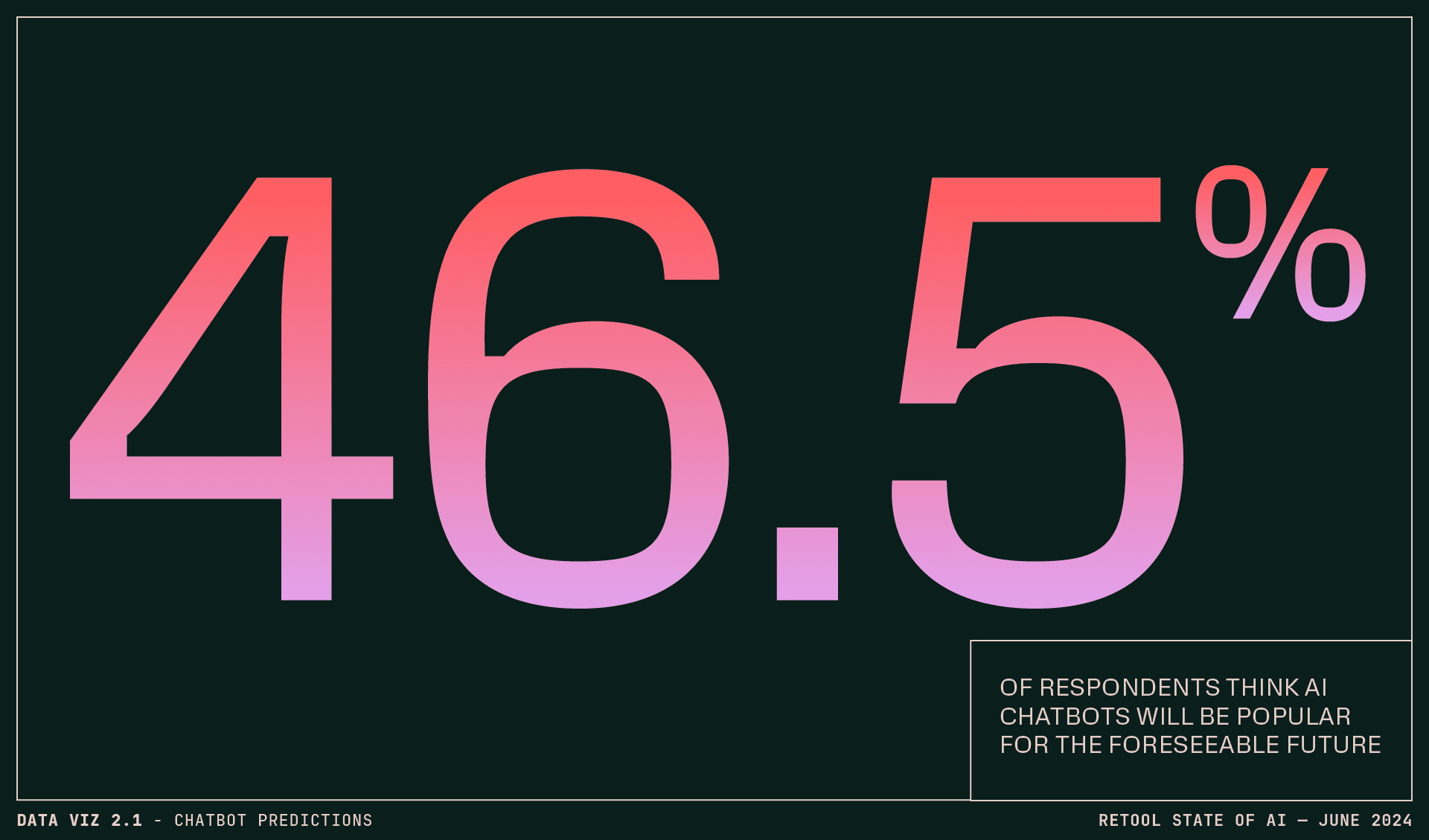
Support chatbots, often a “go-to” use case and example, are only the third-most popular live internal use case for respondents, with writing code or queries in the top spot at 42.1%, followed by knowledge base Q&A at 36.4%. Content generation use cases are a bit of a mixed bag.
FWIW, we kept it old school, and didn’t use AI to generate the content in this report.
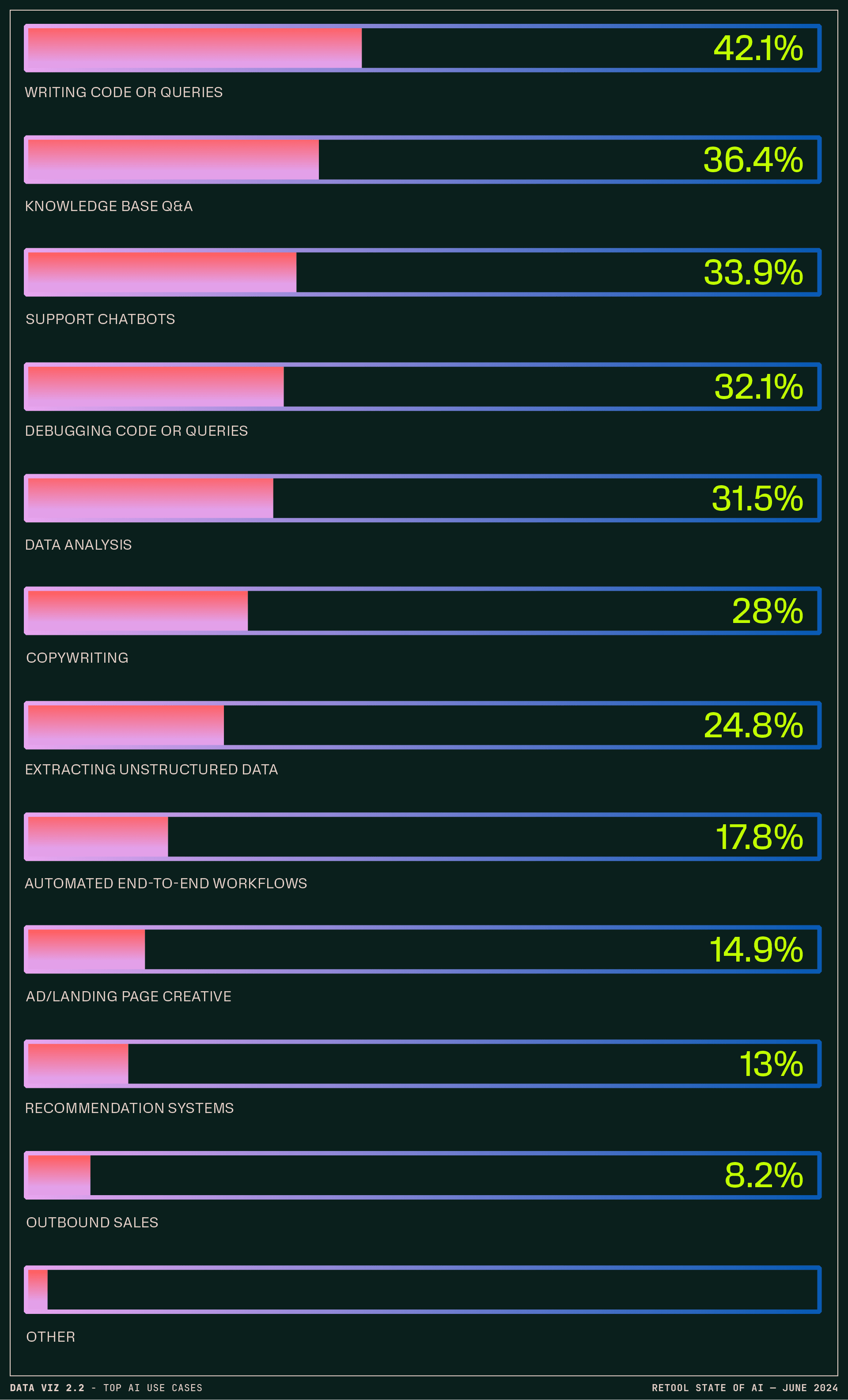
While most use cases were within a few percentage points from our last check in in late 2023, a few areas had about a 5-point swing, trends we’ll keep an eye on:
- Writing code or queries dropped 5.4 points from from 47.5 to 42.1% as did copywriting, from from 32.9% to 28%
- Support chatbots jumped up 5 points, from 28.9 to 33.9%
- Automating workflows jumped up nearly 5 points from 12.9 to 17.8%
We also saw a diverse distribution of AI use across departments.
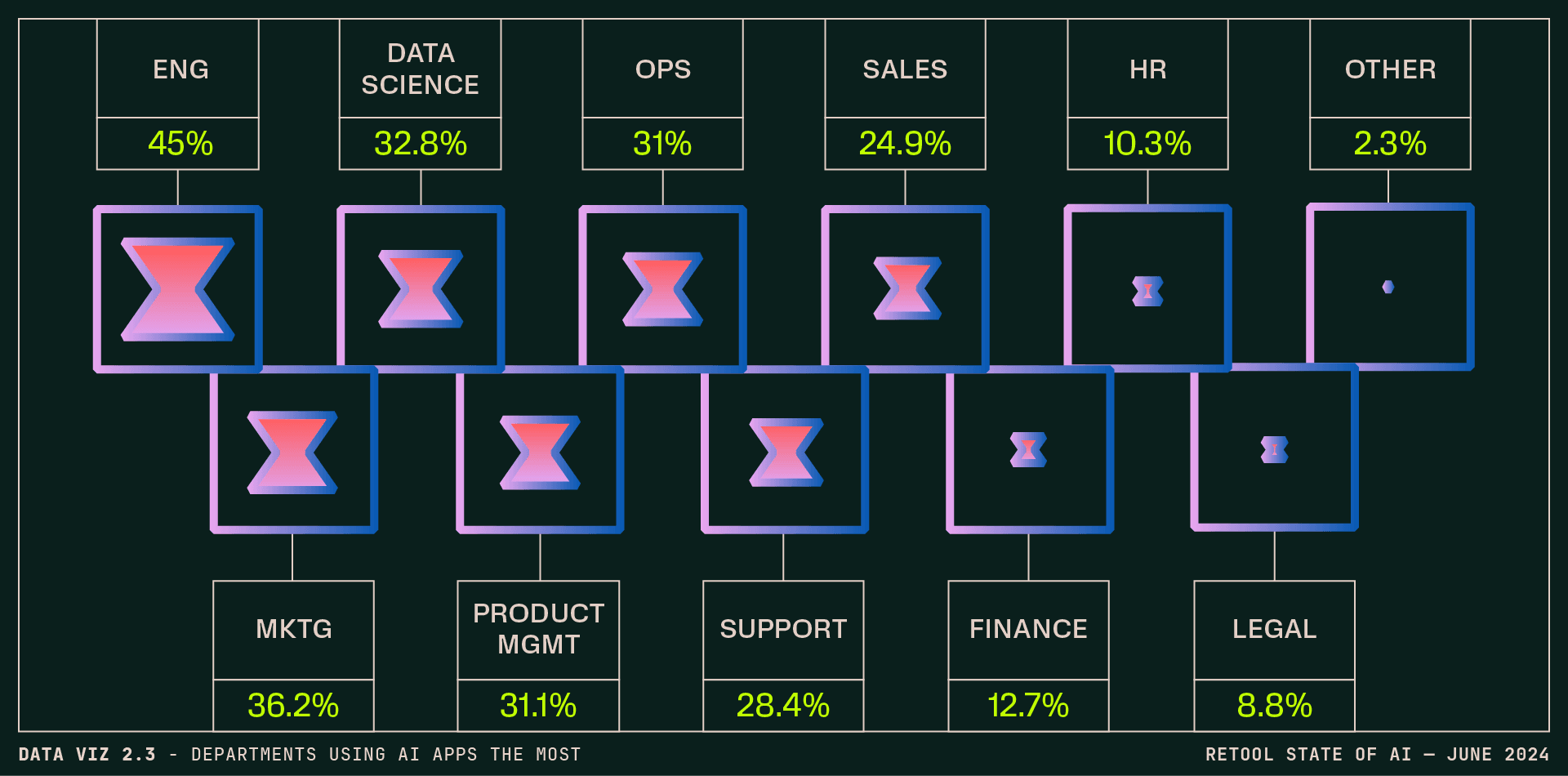
On first blush, it seems contradictory for Support to lag behind other departments in adoption (coming in sixth, after Engineering, Marketing, Data Science, Product Management, and Operations), especially since support chatbots are still a close third for live internal use cases.
Realistically, support chatbots in use and the actual percentage of Support teams using AI-powered apps are just a few percentage points off from each other—but it wouldn’t be unreasonable to see some reticence rolling out AI support to customers:
- Two of the top three pain points respondents associated with developing AI apps were model output accuracy/hallucinations (38.9%) and data access/security (33.5%) which have remained largely unchanged from 2023’s survey.
- Trust in the output of the models was mostly low to moderate across all roles, with an average of 6.1 out of 10 (even if respondents generally liked and wanted to continue using said models!).
Only 8.5% of respondents saw strongly more promise for AI in external use cases, versus 57.9% equal promise for both internal and external, and 33.7% more promise for internal.
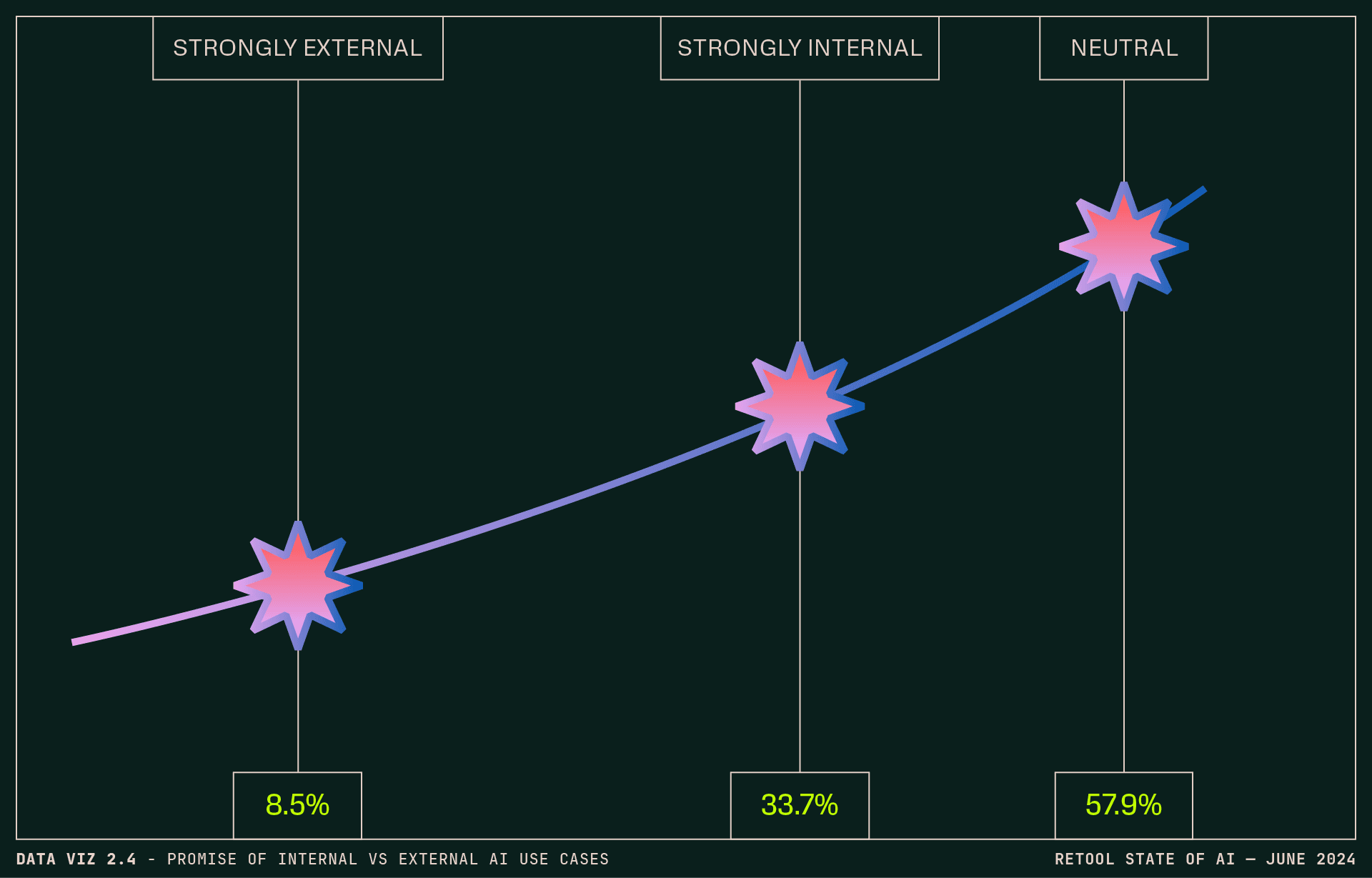
It holds that companies may want to reduce risk and experiment with internal AI apps that enable team members to self-serve support before exposing customers to these risks.
So we know, by and large, the types of AI apps respondents are building, and for who—but what comes next? And what use cases, applications, big swings, or little odds and ends are getting them excited to build with—and just use—AI? We saw some trends in the write-ins.
Overall, some respondents are using the current world of “AI things” with enthusiasm—to assist with generating first drafts and pitch decks, to analyze text, and assist with writing and debugging code—but others commented that the current uses for AI are limited. Writ large, there's an expectation that we'll see new and innovative uses develop as the technology—and our collective ability to build with it—matures.
A look at—you guessed it—the AI stack
As more developers build more AI apps—and explore the most effective ways to do so—we wanted to know more about the tools they’re using to make it all happen. What’s working well in the stack, and what isn’t? How much model customization is going on? Does anyone really have a sense of whether GPUs are being allocated right? Let’s dig in.
OpenAI models are still the most frequently used in production, accounting for around three-quarters (76.7%) of AI users’ models of choice. GPT-4 leads at 45%, followed by GPT-3.5: at 25%. (We didn’t ask about 4o as it was just emerging at the time of the survey, but we’d bet respondents are trying it.) This pattern plays out by sector and company size too, with OpenAI in the lead across the board.
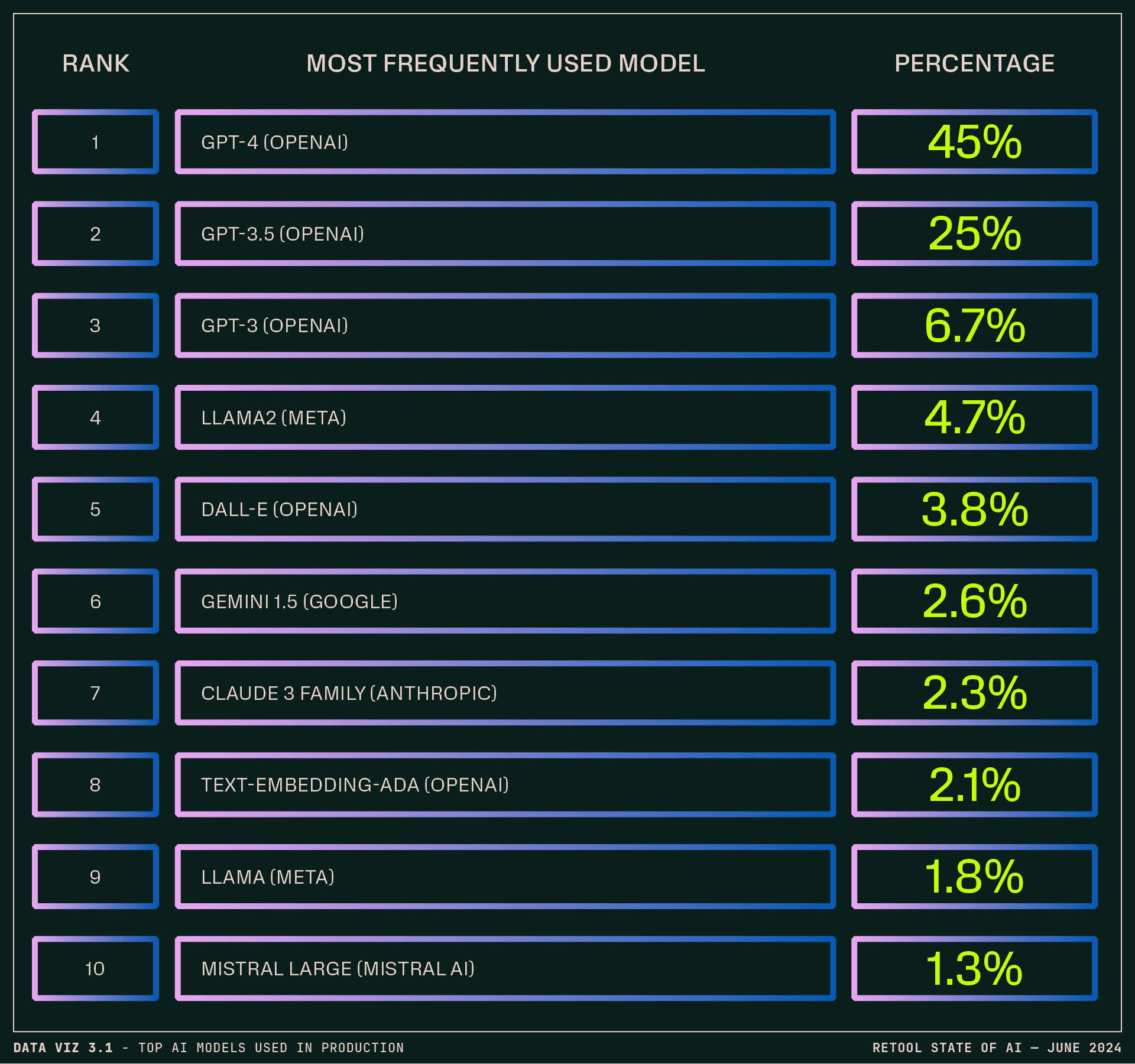
That said, some interesting highlights—the numbers for Anthropic’s Claude 3 alone more than quadruple Anthropic’s showing in our last report. (And that’s not counting earlier Claudes.) Mistral, which recently raised quite a round, emerges on the list as well.
And, sure, we heard some of the common gripes with the various flavors of GPT (“I’d like it to tell me when it doesn’t know something instead of providing false information”), but respondents were pretty satisfied with their model situation. A little more than one-third (35.5%) were very or mostly satisfied; 34.5% were at least somewhat satisfied; 11.9% weren’t really thinking about it one way or the other. Of those who wanted to change something about their AI stack, only 17.1% were unhappy with their model provider; 12.3% were just looking to switch to a different model from the same provider.
A majority of respondents are customizing their models to some extent, with most fine-tuning existing models (29.3%) or using vector DBs or RAG (23.2%). (On the latter, companies over 5000 have a ~10 point lead on the average at ~33%.)
Getting into how people are keeping their apps accurate, teams are using a variety of methods: feedback loops from users are the most popular (39.5%), quickly followed by prompt engineering iteration (36.4%) and model monitoring and evaluation (35.9%).
We also heard general concerns around bias and fairness of models from respondents in their write-ins, and those concerns aren’t unfounded: nearly 30% of respondents reported that they either don’t care or don’t know how to address bias and fairness in their models. (Another 25.9% said they don’t currently address this but want to.) There are layers here: some companies may expect foundational models to build in bias correction already, some bias concerns may appear inapplicable to the types of inputs and tasks they’re using AI for (writing unit tests versus marketing copy, for example), and then… there are some who just genuinely don’t care.😩
Among those who are addressing bias and fairness, the most popular methods are regular audits and reviews (30.4%), preprocessing techniques like data augmentation and bias correction (23.8%), and post-processing fairness adjustments (17.8%).
Digging in further, 17.7% of survey respondents’ companies are building their own models. Notably, financial services companies trail at 11%. Some companies may benefit from custom LLMs by offering advanced data security, adaptability to market shifts, and custom services and tools, but given the high initial costs of training models and ongoing maintenance, it might be surprising to see this many companies going down this path.
That said, there are some pretty clear delineations here:
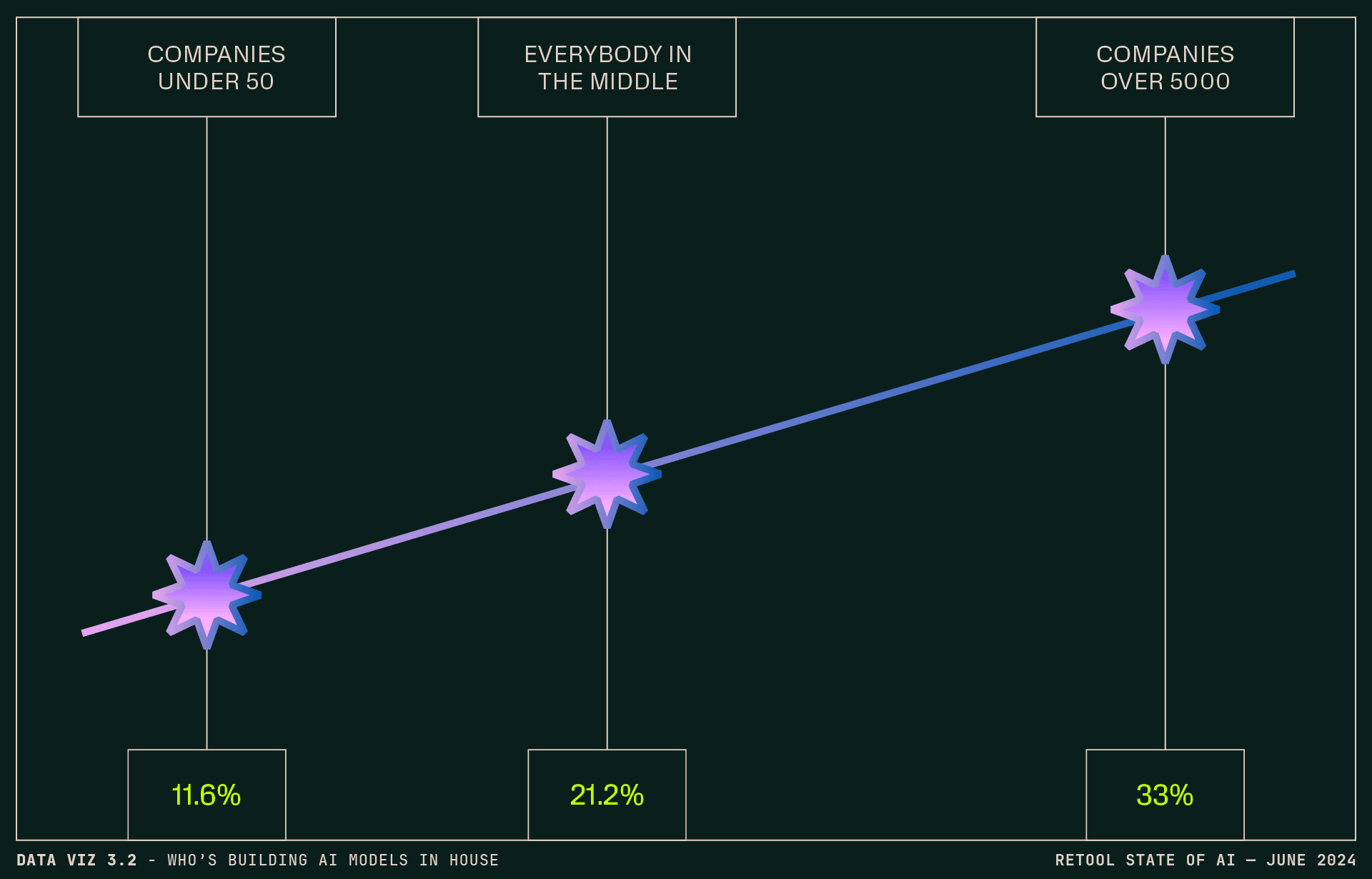
Today, most respondents reported using vector DB (63.6%)—a big leap from 2023’s 20%. (And the more people use their vector DB, the more they recommend it. Which came first, the chicken or the egg database?)
Going one further, those at the biggest companies were the most likely to use vector databases or RAG to customize their models. (33% at companies over 5000.)
Here’s the leaderboard:
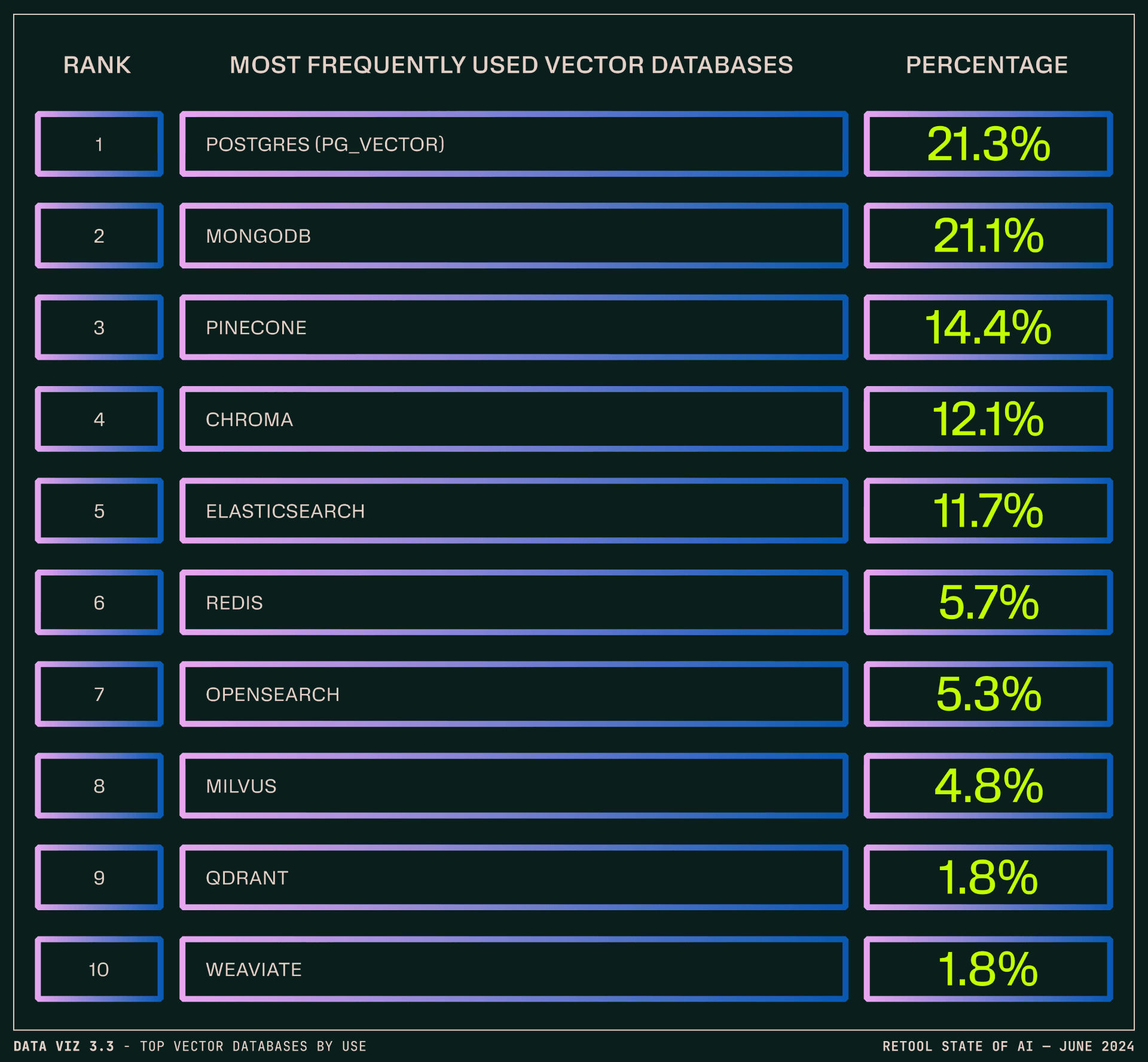
It’s true that using a vector database can introduce a lot more “knobs to turn” in terms of configuring retrieval chunk length, how data is fed to the model, etc.—so it’s worth shouting out those whose regular users were particularly satisfied. MongoDB maintained the strong performance we saw in last year’s report, with the most satisfied regular users as measured by NPS. Chroma climbed the NPS charts, coming in a close second after MongoDB. (Bonus shout out to Qdrant—while the sample size was much smaller, their users were similarly happy.)
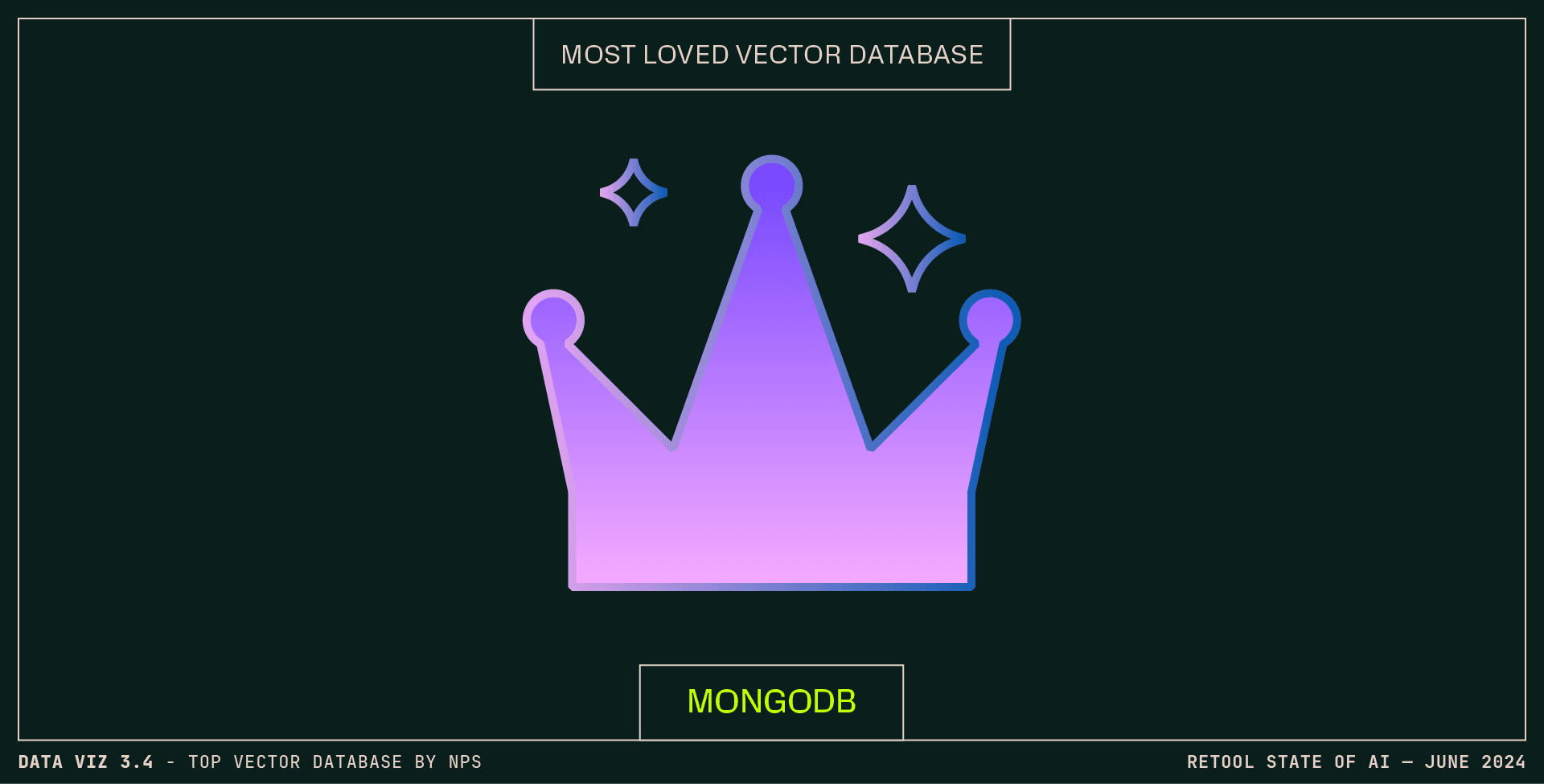
When evaluating and comparing vector databases for projects, the most popular method was using performance benchmarks (40%), followed by user reviews and community feedback (39.3%), and proof-of-concept experiments (38%).
A slight majority of respondents (51.9%) aren’t using an inference platform at all, which may not be so unsurprising given the associated hardware requirements and cost of training. (The second-most reported pain point of developing AI apps was a lack of available technical expertise/resources at 38.2%.)
Of those who are using an inference platform, Amazon Sagemaker (13%) and Databricks (8.6%) were the winners for frequent use, with a fairly even distribution among the rest. (Databricks got the highest marks for user satisfaction.)
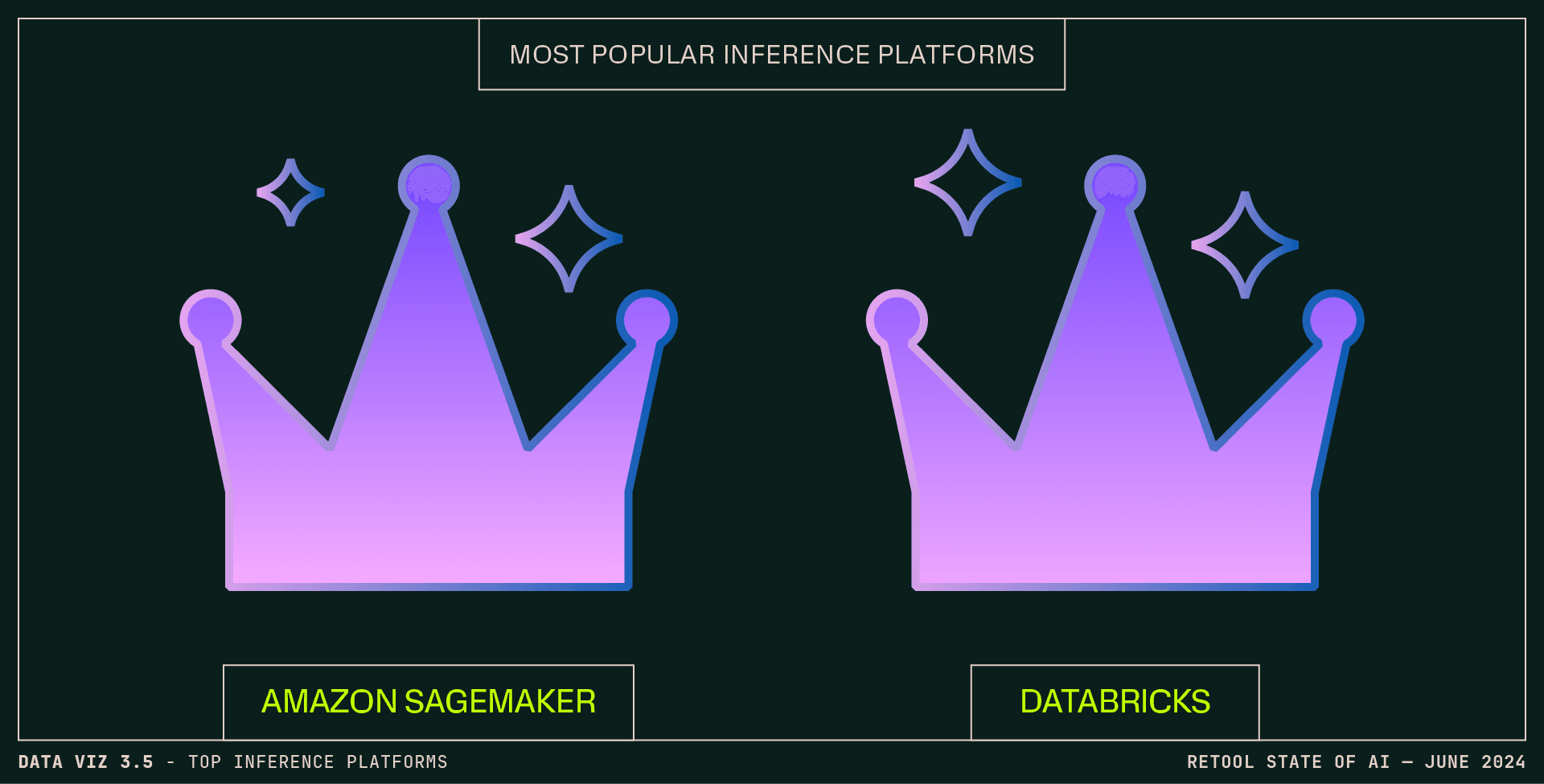
Most companies (59.1%) are using some form of additional tooling for AI development, whether homegrown or off the shelf. (Phew? Phew!) For those reporting use of just one tool, HuggingFace is in the lead—15.8% reported using it (and, proportionately, more l-o-v-e it than any other tool, model, inference platform, or db in the survey!). Custom tools (10.6%) were the second most used solution. (Braintrust was in the ~middle of the pack when it came to usage, but was a strong second in satisfaction.)
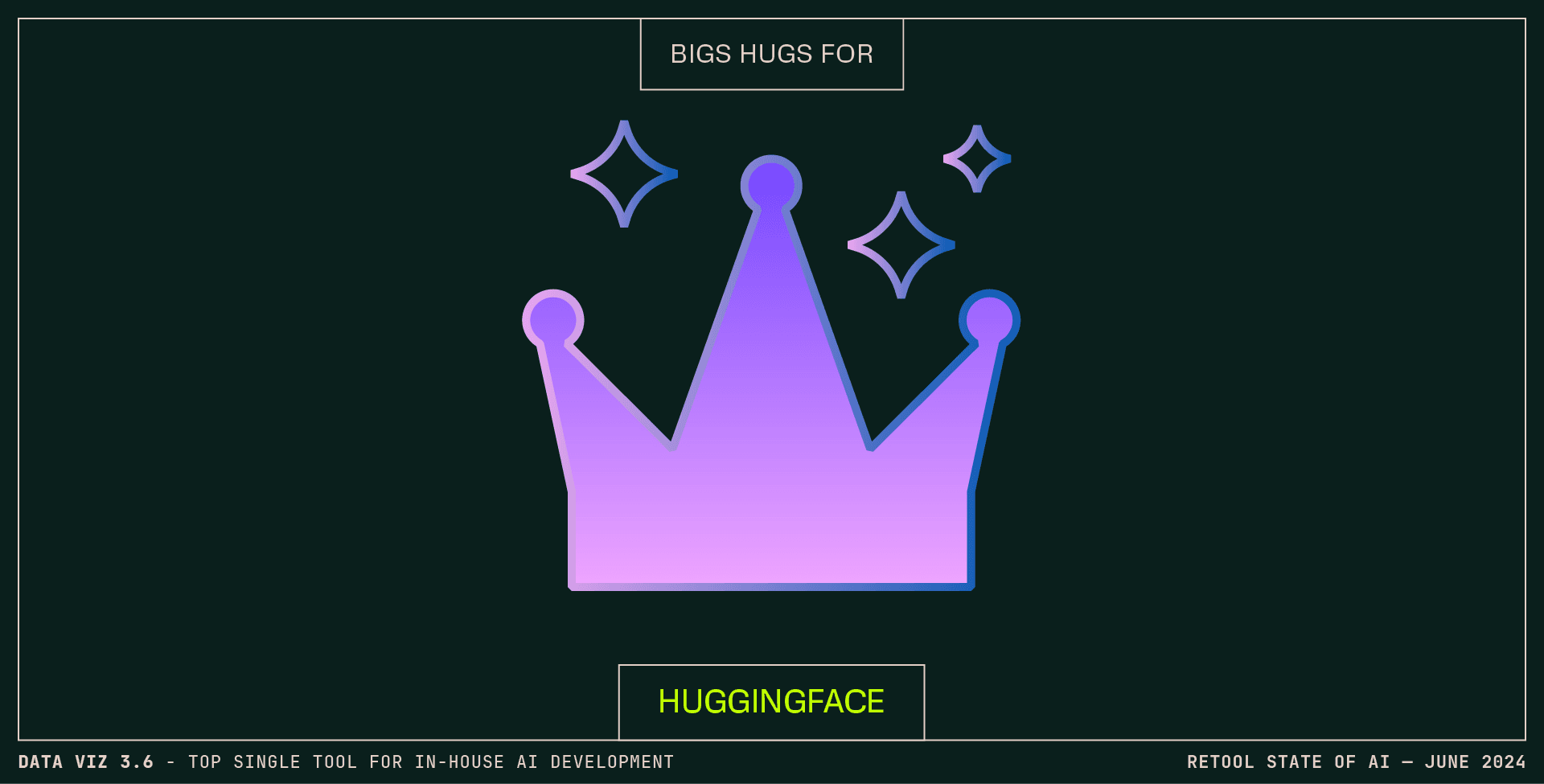
For those using more than one solution, LangChain (21.3%) and HuggingFace (20.1%) were used most often. A slight majority (56%) is using a framework for building UIs.
Bear in mind that 40.9% of respondents aren’t bringing in any additional tooling for AI development—so the landscape is set to shift and settle as teams build out their AI development stacks.
With costs of model training and implementation top of mind for many engineering leaders, we wanted to know how companies are approaching AI hardware and resource allocation. A minority own or operate GPUs themselves (13.2%), with the rest split between renting from major cloud providers (38.9%) and emerging providers (15.8%). Just under a third aren’t using GPUs directly at all.
And the jury is out on whether the operating costs of GPUs (rented or owned) are returning on the investment: a slight majority (53.7%) say yes versus 30.6% that say no or don’t know (5.1% and 25.5% respectively)—perhaps pointing to a challenge of measuring ROI.
That said, investment in GPUs may be consistent with scale: the largest companies (5000+) are most likely to report positive ROI from GPU usage at 40%, with the smallest (companies of 1-9) least likely to find it a happy breakeven or better (19%).
Things get foggier when trying to evaluate how well companies have allocated GPU resources, with respondents divided 47.7% yes to 52.3% no or don’t know:
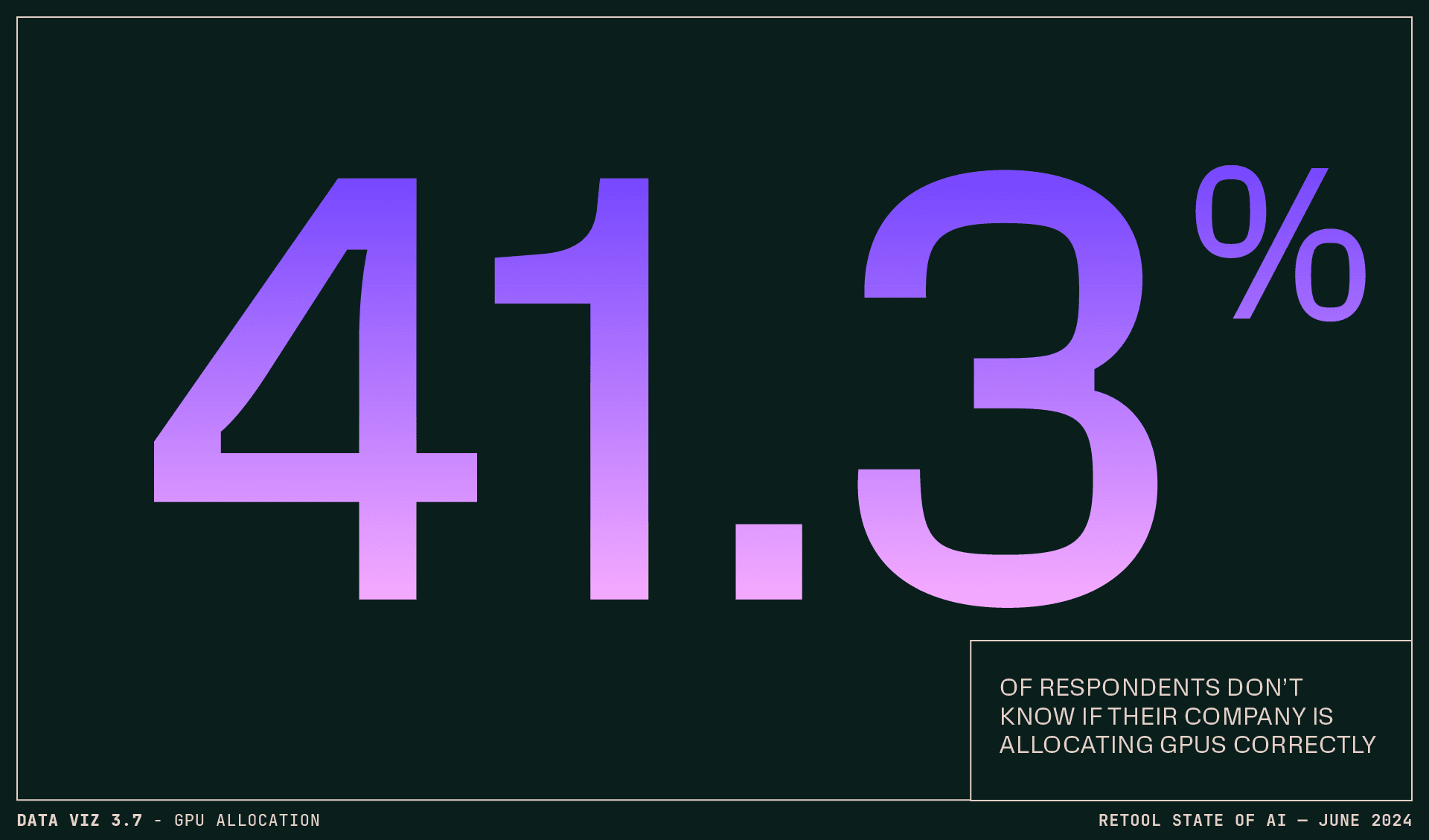
Interestingly, the perceived ROI of GPUs isn't necessarily tied to the perceived “correctness” of allocation. Almost half of respondents (46.8%) who answered "no" or "I don't know" when asked about their company's GPU allocation still reported seeing at least some ROI from their company's GPU investment. 🤔
Respondents who feel their company allocates GPUs correctly (72.7%) are more likely to report seeing positive ROI on their GPU investment. Consistent reevaluation of GPU allocation might be in the cards as the AI stack continues to evolve.
It’s not all sunshine and roses, but there is sunshine, there are roses.
We asked about the biggest pain points developing AI apps:
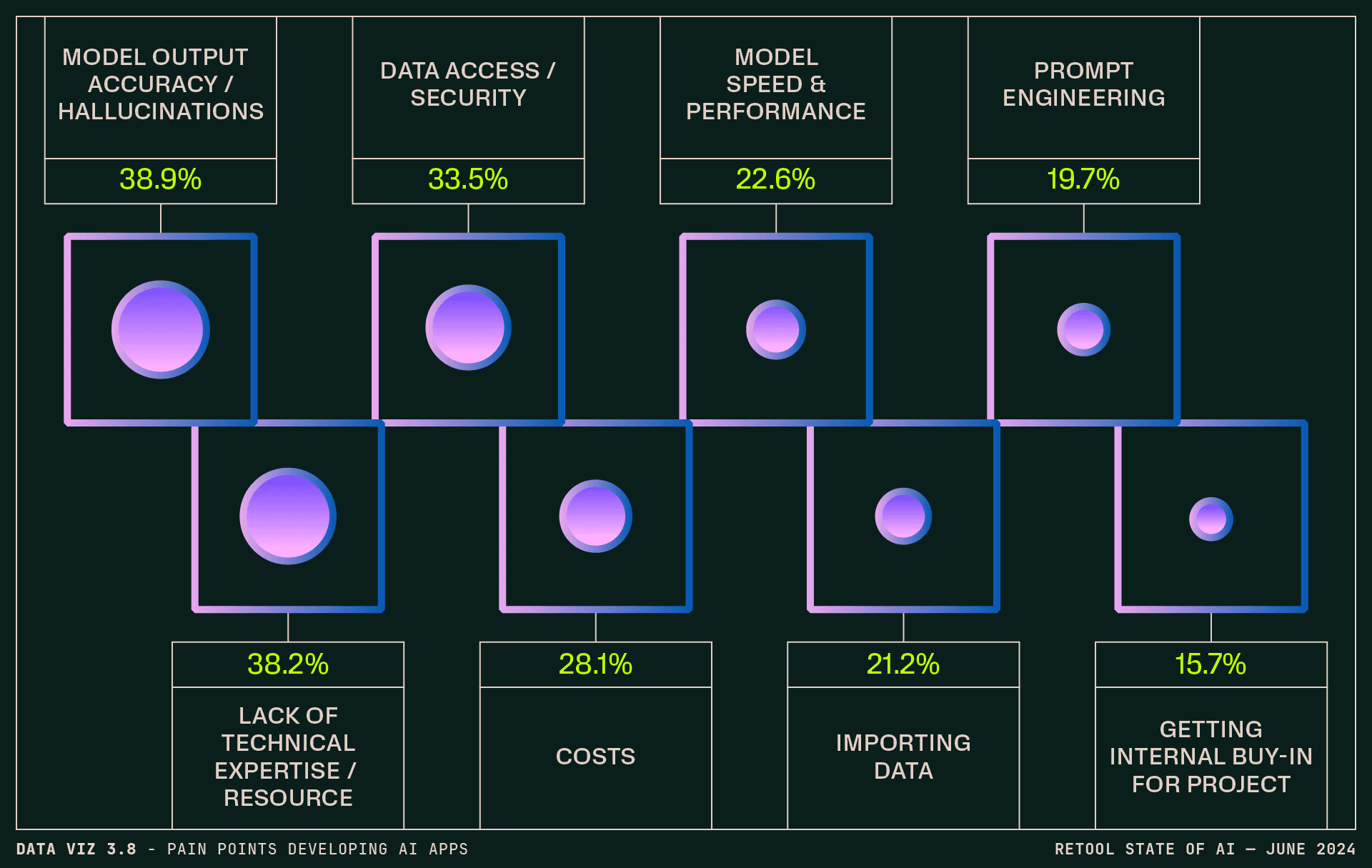
And, at least for the moment, respondents are finding areas of the AI stack where they’re more settled—and areas that leave room for improvement.
Overall, respondents are more satisfied with their stack than not:
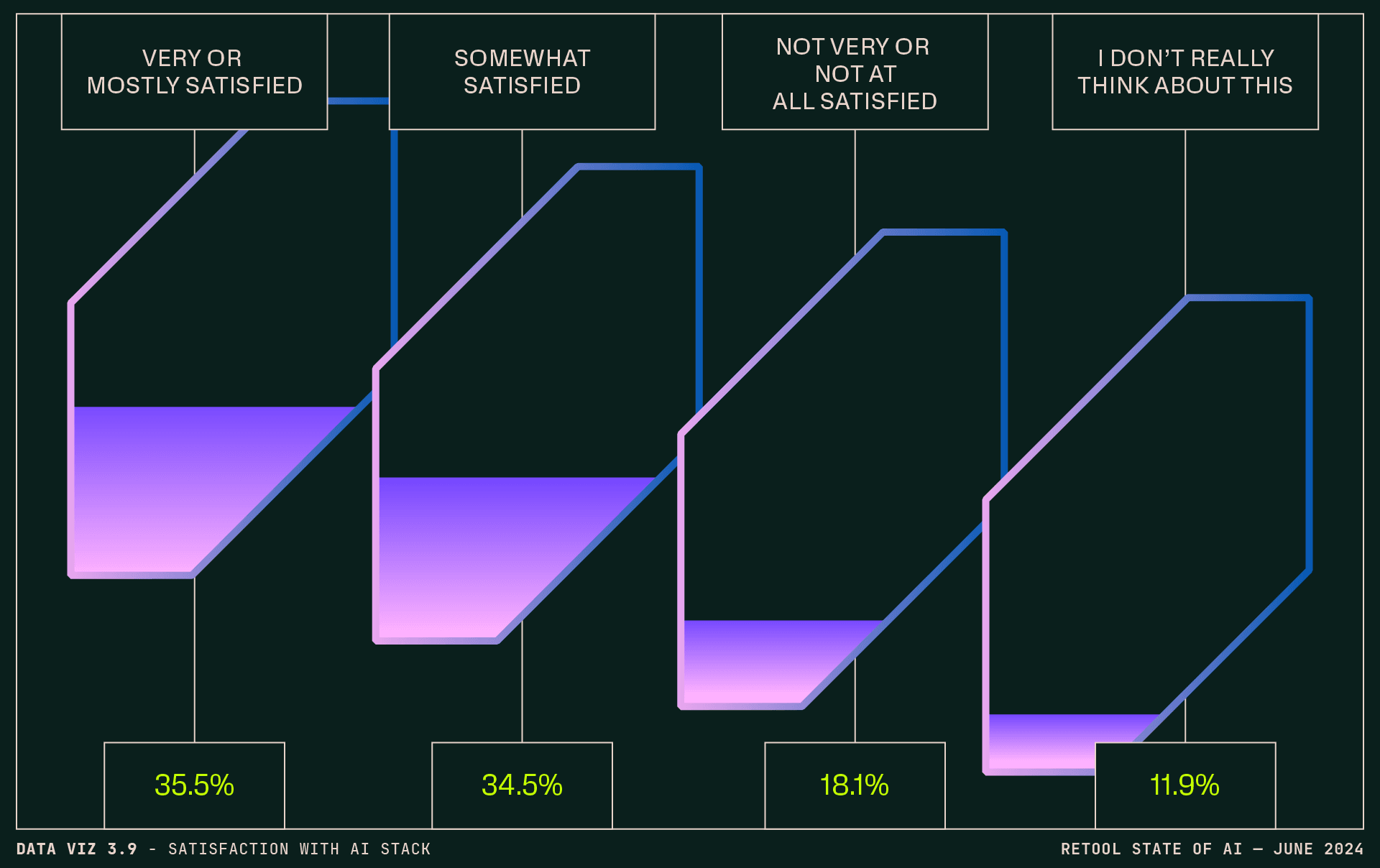
And at the poles, more than twice as many (10.2%) are very satisfied than want to completely start over (4.5%).
By industry, respondents in the Healthcare (45%), Education (43%), Consulting (42%), and Non-profit sectors (40%) were most likely to say they were very or mostly satisfied with their stack. (The Materials sector also ranked highly, with the caveat the sample size was notably smaller.) The Energy (15%) and Government (21%) sectors trail significantly. By company size, all companies fell between 30–40%, with no outliers.
Moving along: folks mostly (72.1%) know what they need to change, and sometimes that’s multiple things:
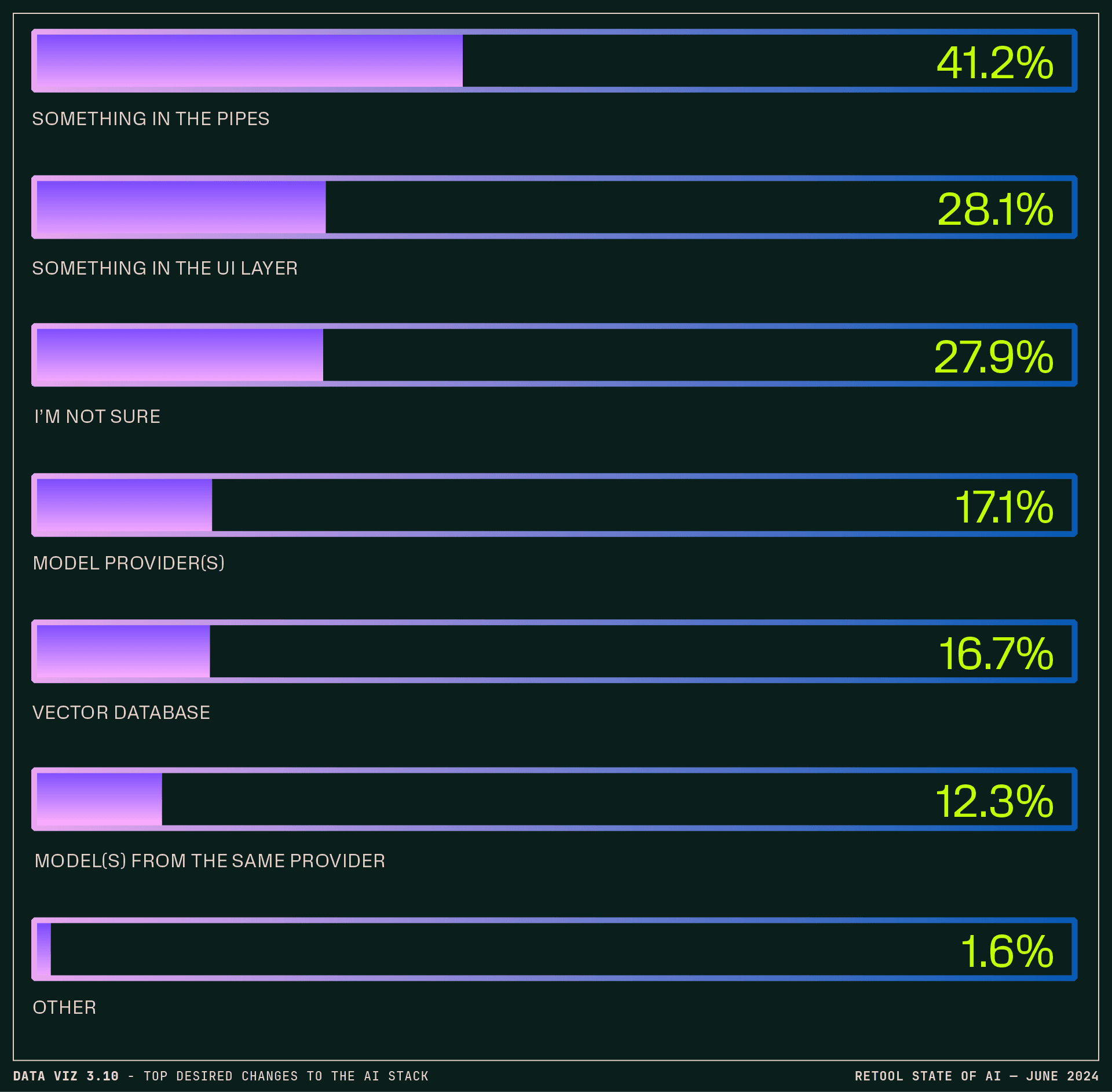
Seems like there’s some work to be done in the middle of the stack—and that, while things are looking decent overall, more flexibility around switching between tools and models might enable those building with AI to get the results (and satisfaction) they’re looking for.
For a few more thoughts on what comes next, respondents shared their hopes, dreams, and expectations of how the AI stack will evolve:
It’s clear that on an individual level, AI has a meaningful impact on the way people work, whether that’s IC developers, product teams, or senior leadership. It’s also clear that businesses are still figuring out how to make the most impactful use of the evolving tech. But there are signs pointing toward maturation and refinement in what developers are building and focusing on, and how folks across the board are thinking about the role of AI in production.
Taken all together, maybe there’s the start of a roadmap, or at least some indicator lights, for how leadership can help developers enter the next chapter. Developers need more than just better AI tech and tooling to build AI apps with real ROI—they need executive buy-in, adequate staffing and funding, and robust data governance to succeed with AI projects.
There’s an opportunity to lead by example, to get clear about expectations around AI, and to make sure developers are resourced with the right tools to build, iterate, and learn quickly.
To end on a curveball, let’s get existential for a minute.
59.9% of respondents think the likelihood of AGI in the next 10 years is high, very high, or certain. 2.7% think it already exists. What will the chatbots think of that?
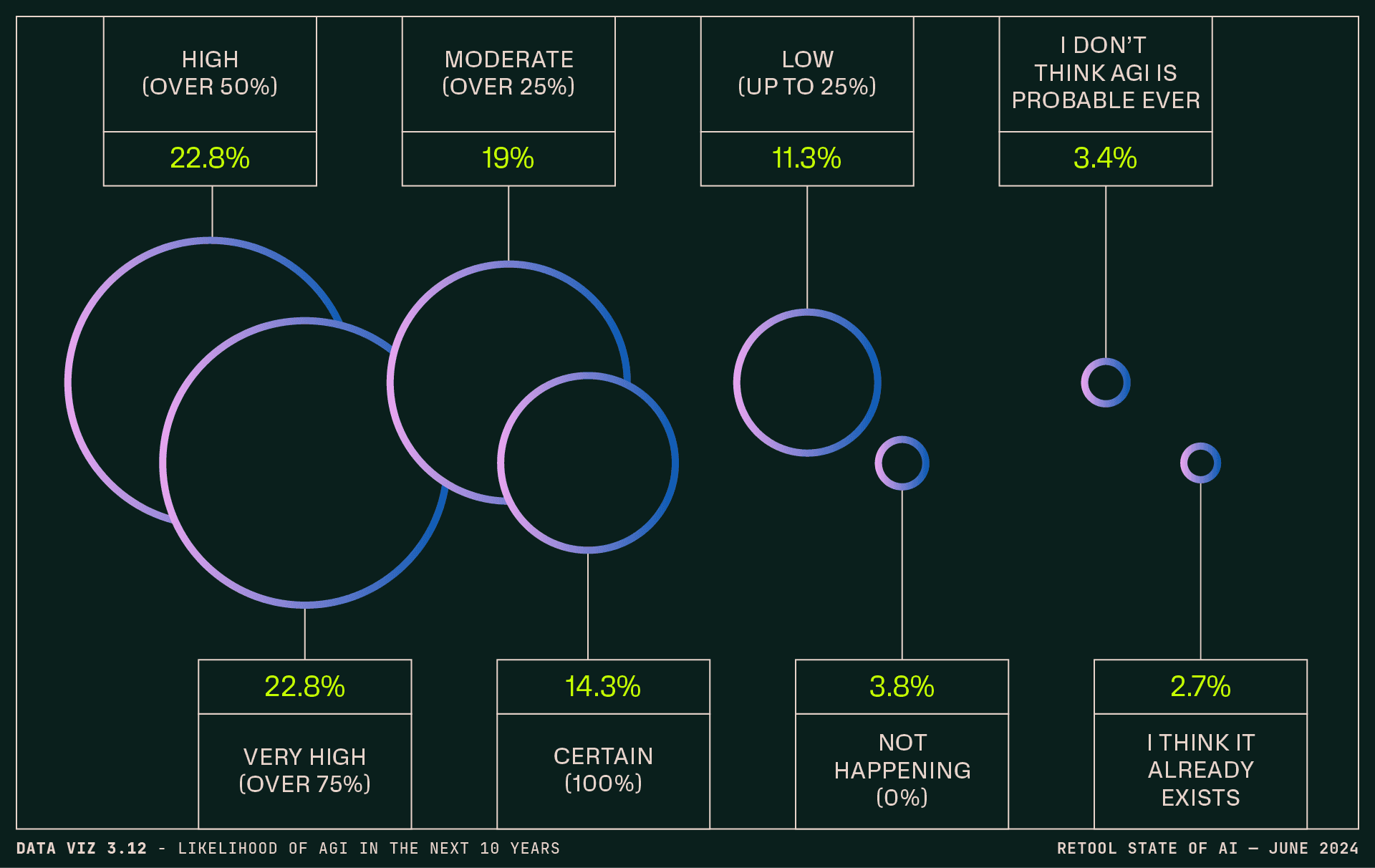
--
The insights in this report were gathered from a public survey of 730 people in April 2024. Top 5 industries: Technology (37.3%); Consulting/Professional Services (9.5%); Financial Services (7.8%), Media/Communications (7.2%), Education (6.6%) || Top 5 teams: Engineers (31%), Ops (19.5%), Product (15.4%), Data (11%), IT (10.9%) || Top 5 roles: Mid-senior level (30.8%), Director/Manager (30.1%), C-suite (17.4%), Entry level (12.4%), VP (6.4%) || Breakdown by company size: 1-99 employees (59.3%), 100-999 employees (28.1%), 1000+ employees (12.6% of respondents)
Content & Design: John Choura, Rebecca Dodd, Willa Gross, Matthew Isabel, Keanan Koppenhaver, Kelsey McKeon, Nate Medina, Sid Orlando, Justin Pervorse, Mathew Pregasen, Cam Sackett, Chris Sandlin, and more.